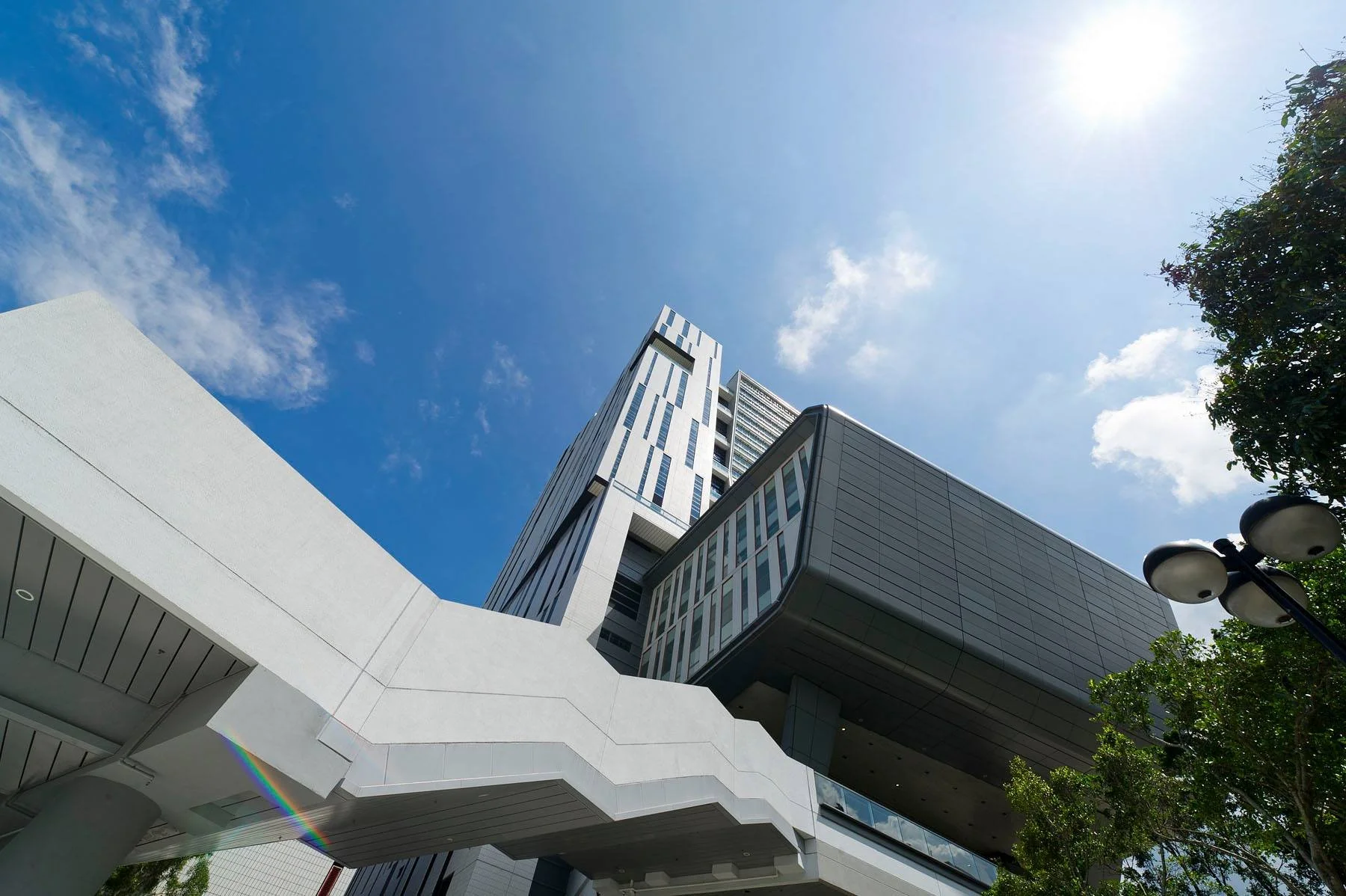
Functional Adaptive Double-Sparisty Estimator for Multivariate Functional Linear Regression Models
25 skeletal joints captured by a Kinect sensor
Timed Up and Go Test
The estimated coefficient functions of vertical velocity for different joints. Each curve represents the estimated coefficient function of a joint and is color coded based on its position of body skeleton.
We have been developing a new multivariate functional linear regression model with one-stage double-sparsity estimation to simultaneously achieve global sparsity in functional covariate selection and local sparsity in coefficient estimation with zero sub-regions. The method is practically appealing to analyze multiple signals monitored by sensor devices. For example, we applied our method to assess the association between multi-joint movement patterns captured by a Kinect Sensor and elderly physical health assessments.
Deep Learning and Explainable AI for Dementia Prediction
Raw MRI image
Heatmap
MRI with Heatmap
Dementia-related conditions cause significant cognitive impairment and are often economically and socially burdensome to society. Accurate prediction of future cognitive decline can allow for interventions that slow neurodegeneration and improve health outcomes. Our work has focused on using deep learning to develop explainable prediction models for dementia using MRI data.
Medical Image Translation with An Application in Immunostaining
Basic model framwork
Hematoxylin and eosin (H&E) staining is the gold standard for diagnosing a range of histopathologic conditions, however, it can be fairly time intensive. Our lab has been focused on training neural networks on H&E imaging data to develop a model that can perform the staining procedure virtually. In addition, we are also interested in how explainable AI techniques can provide practical information to clinicians during the staining process.
Clinical Prediction Models in Geriatrics
(A) Daily activity taken from smart watches worn by elderly residents in the United Kingdom
(B) A partial dependence plot showing how our depression screening model predicts depression risk for varying levels of life satisfaction among elderly Chinese
As the global population ages greater attention is being given to personal health monitoring, predicting patient outcomes, and early disease detection in elderly populations. We have a keen interest in developing prediction models in geriatrics, particularly for psychiatric disorders and neurodegenerative diseases. Our recent work has included depression screening in China and suicide prediction in the United Kingdom. Additionally, we have been exploring if objective activity measurements from wearable devices can improve prediction models for dementia and suicide.
Integrating Gene and Wearable-Device Data for Early Parkinson Prediction
Basic model framwork
We propose a multi-modal deep learning model that integrates wearable device data and genetic data for early prediction of Parkinson’s disease, up to 7 years before clinical diagnosis. While existing studies focus on symptom detection, early prediction remains underexplored. We design accelerometer encoder to process long-sequence movement data, a gene encoder to extract features from significant GWAS SNPs, and a cross-attention fusion module to combine both modalities. We also introduce knowledge distillation to enable high accuracy using wearable data alone, enhancing real-world applicability.
Leveraging Wearables in Aging Research
Figure 1. The ability of wearable-based machine learning models to predict poor performance on cognitive tests in the NHANES (Sakal et al. 2024, published in npj Aging)
Figure 2. Associations of pre-bedtime physical activity timing with poor sleep quality among adults in the UK Biobank (Sakal et al. 2025, published in SLEEP).
Wearables capable of tracking lifestyle and physiological health markers are increasingly recognized as key to gaining a deeper understanding of healthy aging. In our research, we leverage large population cohorts such at the UK Biobank and NHANES to examine how objectively measured sleep, physical activity, and circadian rhythms are associated with adverse health outcomes in older populations. We also examine how wearable device data can be used to train machine learning algorithms for personal health monitoring, such as predicting cognitive function, Parkinson’s, and forecasting sleep quality before bedtime.
Multi-Modal Survival Prediction with Deep Learning
Model framwork for multi-modal survival prediction
Accurate survival prediction for cancer patients plays a vital role in informing therapeutic strategies and optimizing clinical decision-making. In this work, we propose multi-modal frameworks that aim to improve survival prediction by enabling effective representation learning and integration across diverse data modalities.
Multilevel Functional Dynamic Regression with Application in Sleep Efficiency
The plots show the 2D relationship between sleep efficiency and physical activity level within one week. Large coefficient value corresponds to better sleep quality.
We have been developing a multilevel functional dynamic regression model to capture the longitudinal trajectories as well as the multilevel structure exists in the dense and regular repeated sampled functional data. The model can be applied to explore the relationship between sleep efficiency and daily physical activity profiles derived from accelerometer datasets.